Freemium Customer Conversion to Pay
Drilling Down
Newsletter # 112: 10/2010
Drilling Down - Turning Customer
Data into Profits with a Spreadsheet
*************************
Customer Valuation, Retention, Loyalty, Defection
Get the Drilling Down Book!
http://www.booklocker.com/jimnovo
Prior Newsletters:
http://www.drilling-down.com/newsletters.htm
-------------------------------
Hi again folks, Jim Novo here.
We're talking about the Freemium model again, in particular, can
you optimize the front end of the business to generate trial accounts
that are more likely to convert to paid?
Answer: Of course you can!
Can you use a "cloud-based" web analytics tool to do
this?
Answer: Probably not without a ton of pain, but you can do some
simple offline analysis first that will enable you to reduce the pain.
We're back in Customer LifeCycle land, using the behavior of
customers today to tell us what they are likely to do tomorrow.
But we can't use a simple RF model for this one - might just have
to create a simple graphical trend model and "eyeball" the data
for clues.
It's Visitor Analysis meets Customer Analysis (or perhaps Web
Analytics meets BI) - are you up for some cross-mode Drillin'?
Questions from Fellow Drillers
=====================
Freemium Customer Conversion
Q: I was wondering if you've done any work with, or given thought to, companies who have a cloud based Freemium business model?
Should they be tracking usage (or anything) at the free level?
Should they be tracking usage at the paid level? I'm sure defection rates
are a big problem, but I'm wondering how many focus on engagement thru mass marketing versus trying to keep what they've got, or influence the free users to make the leap to paid.
Any thoughts on this? Maybe you could do a blog post on it.
It seems like a good fit with your brand of analysis but I'm just starting to think it through...
A: I just finished an analysis that's a good example of
this problem. Behavior during the Freemium period can predict who is highly likely to become a paying customer, who will need marketing efforts like additional sampling / package discounts, and who will not become a customer no matter what you do.
So the answer is you need both, analysis of paid and free. But in particular, what
you need to do is understand the transition from free to paid by
comparing the behavior of known converters versus non-converters
over time, preferably using events that create value for customers.
Typically the differences will be volume / persistence related, generically, low Recency high Frequency.
Also, likely converters to paid will tend to use a wider variety of features consistently.
In the analysis, the question to answer is which of these
value-creating behaviors is predictive of becoming paid?
Said another way, you tend to see a fairly fast drop-off among *all* new Freemium customers after the initial burst of activity, but the ones that are not going to stick tend to drop off even faster in their activity.
Then, there is a "bounce" in activity where the ones who are most likely to end up as paid begin to cycle behavior more quickly and begin to use more features, and the others simply drift off the map, with no "bounce" as Recency becomes extended.
Classic LifeCycle analysis - customers tell you what they will become in the future by what they do today.
Having the very detailed behavioral information typically seen with interactivity just multiplies the ability to do this kind of prediction.
More on the Freemium model, including determining appropriate cost to acquire,
is here.
Q: Do the standard analytics packages allow a business to look back at the "free" behavior of paid subscribers?
I'm thinking of Freemium cloud based solutions and how they would track this.
Do products like Crazy Egg get you there or do you really need something more
sophisticated to do this kind of analysis?
A: I've never used Crazy Egg so I don't know about that one specifically, but in general you can do quite a lot with the basic
tools that support customizable segmentation.
The challenge with going that way is you have to be super-technical with the implementation to capture
important event data points, you have to create many different segments, and then the killer problem - you can't "re-analyze" a
different approach with these tools, for the most part. If
that's what you mean by "look back", it's highly unlikely
you could accomplish what you need to do.
So it's possible, but these tools are not really designed for "behavior over time" work and certainly don't allow for
much "exploration" of the data - any change in analytical approach is likely to be a "going forward" type of
measurement, not looking back. So there would be lots of iteration before you even knew if the analytics set-up was correct or what events are meaningful.
In other words, it's possible but could waste a LOT of time.
I'd much rather find the system that contains the key elements of activity - when did they sign up, what features are they signed up for, when did they add other features.
This data probably resides in whatever system manages the account.
Dump that data off into a spreadsheet or database, try to figure out what's meaningful,
look for correlations.
Then, once you have a grip on some solid ideas, then maybe you go into the front end and try to align traffic and behavior with known "events" that seem to predict upgrade to pay, if that's what the mission is.
Otherwise, you will be setting up a ton of tracking on all kinds of events not knowing what is meaningful, and then dealing with a really poor interface for the analysis of those events.
The other way to go, of course, is to use one of the advanced web analytics tools, which sit on real databases and can be queried.
But assuming that's not an option, I would try to look for hard data points in the backend first, then knowing key behaviors, look for what might cause those behaviors
in the traffic side.
Example
Let's say you have a project management application that has a 60-day free trial then converts to paid.
Value is created for the customer when they use the functionality of the app - say create project, comment on project, upload
file, or any other actions you deem critical. "Traffic" in a situation like this may be only marginally indicative of value creation;
rising activity could even be a negative indicator (frustration with
application).
So, you want to create a situation where you can analyze these important behavioral events by account, and (ideally) you want to know the source of the account creation - campaign code, referrer, etc.
That's all you need for data, simple table, maybe a dozen columns.
Then, compare average account that converts to paid with average account
opened at the same time as the converters but does not convert,
over the 60-days before trial end. For each of converting and non-converting, aggregate each of
key events by week, divide by number of accounts to get average
behavior per account, and you would have 8 weekly
average data points for each of the events, both for non-converting
and the converting accounts. Maybe a dozen simple line graphs with 8
weekly data points, one set for accounts that paid, one set for
accounts that did not.
Converting and non-converting graphs should look different for some
variables.
Both will typically start out with high levels of activity, then for some
variables you will see them diverge.
This not only predicts which variables affect conversion, but reveals to you the best time during the 60-days to intervene with surveys, help, or other marketing programs to re-engage the accounts that appear to be headed for defection.
If you have campaign data, also which campaigns tend to create accounts that convert and which don't.
One of the event graphs may look to be more predictive than the
others, with abrupt changes in direction going into the conversion
event. For example, perhaps it will look like this:
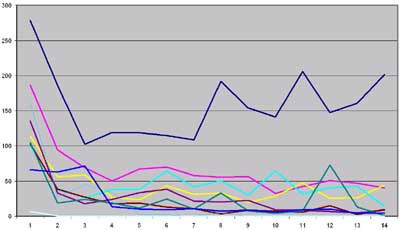
(Click pic for larger image)
This is the behavior of 10 different LifeTime Spend levels (deciles) over
the first 14 weeks of their Life, engaging in an event that
creates value for them. The dark blue line represents average
top spender.
Note how for top spenders, the profile is quite
different. The graph tells you that by week 4 or so, you can
probably predict who will become a best customer and who will need
intervention based on this activity.
You can run this kind of event profile for any
variable - events, campaigns, etc. as long as you know complete /
non-complete goal or end value
of the customer. In your case, there
would be 2 lines instead of 10: converters versus
non-converters. Create a converter versus non-converter chart
for each activity variable and look for this kind of divergence.
Drilling down more deeply by excluding all but 3 lines
so we can see the behavior "in the middle", we find some
interesting patterns:
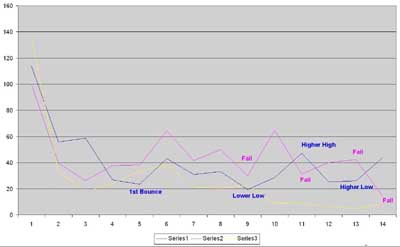
(Click pic for larger image)
Here, we see patterns that provide clues to the
testing targets one might want to address to see if "middle"
customers could be turned into better customers. The blue
segment, showing a series of higher highs and higher lows after it
"bottoms" for this behavior, is most likely to benefit from
intervention of some kind. The pink segment looked promising,
but then put in lower highs and lower lows - these customers lose
momentum quickly and have trouble self-sustaining. The yellow segment was never really in the game
at all.
If I had to pick the segment with the best potential,
I would try the blue segment first, and the data points could be used
for automated triggering of different types of campaigns. For example,
"If by week 4 activity for Variable X falls below 60,
trigger Campaign A. Then if by week 11 activity for Variable X is
not above 40, trigger Campaign B." Remember, these are
averages, so not all customers in the segment are below
threshold. The idea is to target a specific behavior with a
specific message.
Just to be clear, you don't need the goal value of the
customer to put a model into practice, only to prove the initial model
- certain patterns in behavior predict high value customers.
If you want to go further, you could show
these graphs and data to a modeler and see if they can create a more
precise mathematical model, which can be developed much more quickly
with this kind of evidence to review.
Once you fully understand what this LifeCycle landscape looks like, THEN you could go back and instrument the web site and analytical tool to monitor some version of this data in a more automated way.
But trying to guess what's going to be important beforehand and work through a study like this using a vanilla web analytics tool is the very,
very long way to get where you need to go!
Jim
Have a question on Customer Valuation, Retention, Loyalty, or Defection?
Go ahead and send it to me here.
-------------------------------
Need help with creating action-oriented customer analysis or High ROI
Customer Marketing program designs? Click
here.
-------------------------------
That's it for this month's edition of the Drilling Down newsletter.
If you like the newsletter, please forward it to a friend! Subscription instructions are top and bottom of this page.
Any comments on the newsletter (it's too long, too short, topic
suggestions, etc.) please send them right along to me, along with any
other questions on customer Valuation, Retention, Loyalty, and
Defection here.
'Til next time, keep Drilling Down!
- Jim Novo
Copyright 2010, The Drilling Down Project by Jim Novo. All
rights reserved. You are free to use material from this
newsletter in whole or in part as long as you include complete
credits, including live web site link and e-mail link. Please
tell me where the material will appear.
What would you like to
do now?
Get
the book with Free customer scoring software at:
Booklocker.com
Amazon.com Barnes
& Noble.com
Find
Out Specifically What is in the Book
Learn Customer
Marketing Models and Metrics (site article
list)
|